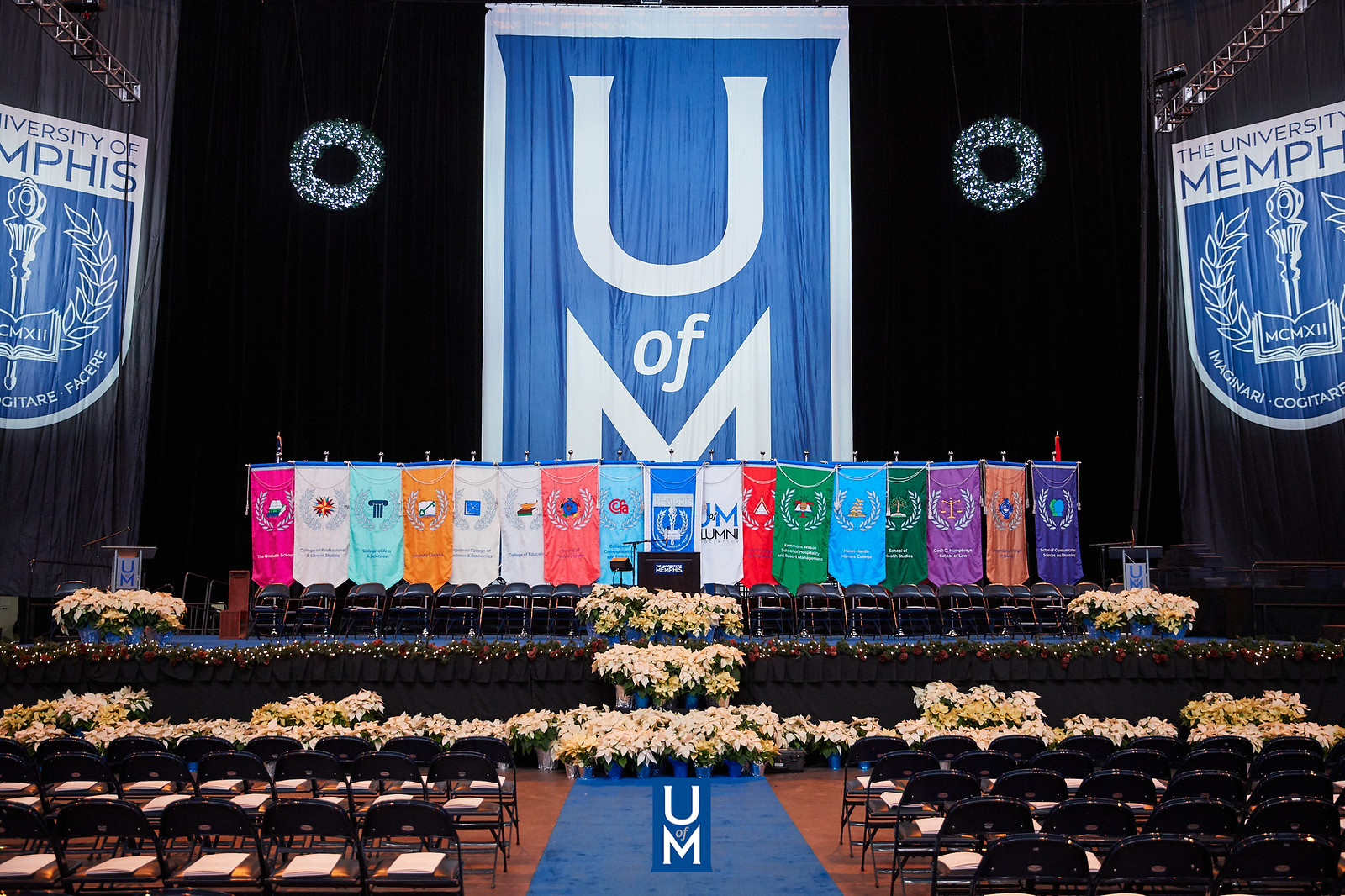
Electronic Theses and Dissertations
Date
2024
Document Type
Thesis
Degree Name
Master of Science
Department
Mathematical Sciences
Committee Chair
Ebenezer George
Committee Member
Baris Kopruluoglu
Committee Member
Majid Noroozi
Abstract
This thesis introduces a novel sequential approach for calculating Bayesian P-values, addressing limitations of traditional frequentist methods. Using Monte Carlo simulations, we investigate the properties of sequentially calculated Bayesian P-values under various conditions, comparing them to frequentist counterparts. Our findings demonstrate advantages including incorporation of prior knowledge, continuous evidence measurement, and adaptability to accumulating data. We explore computational challenges, propose optimized algorithms, and address the impact of prior specification, providing guidelines for choosing appropriate priors. This research contributes to the ongoing discussion about P-values in scientific inference and offers a practical framework for researchers adopting Bayesian methods in sequential analyses. The proposed approach has potential applications across various disciplines, particularly where data is collected sequentially, or early decision-making is crucial. Our work bridges the gap between frequentist and Bayesian methods, offering a more nuanced tool for statistical inference.
Library Comment
Dissertation or thesis originally submitted to ProQuest.
Notes
Open Access
Recommended Citation
Anamege, Johnpaul Chiemelie, "A Sequential Approach to Estimating Bayesian P-values through Simulation Studies" (2024). Electronic Theses and Dissertations. 3595.
https://digitalcommons.memphis.edu/etd/3595
Comments
Data is provided by the student.