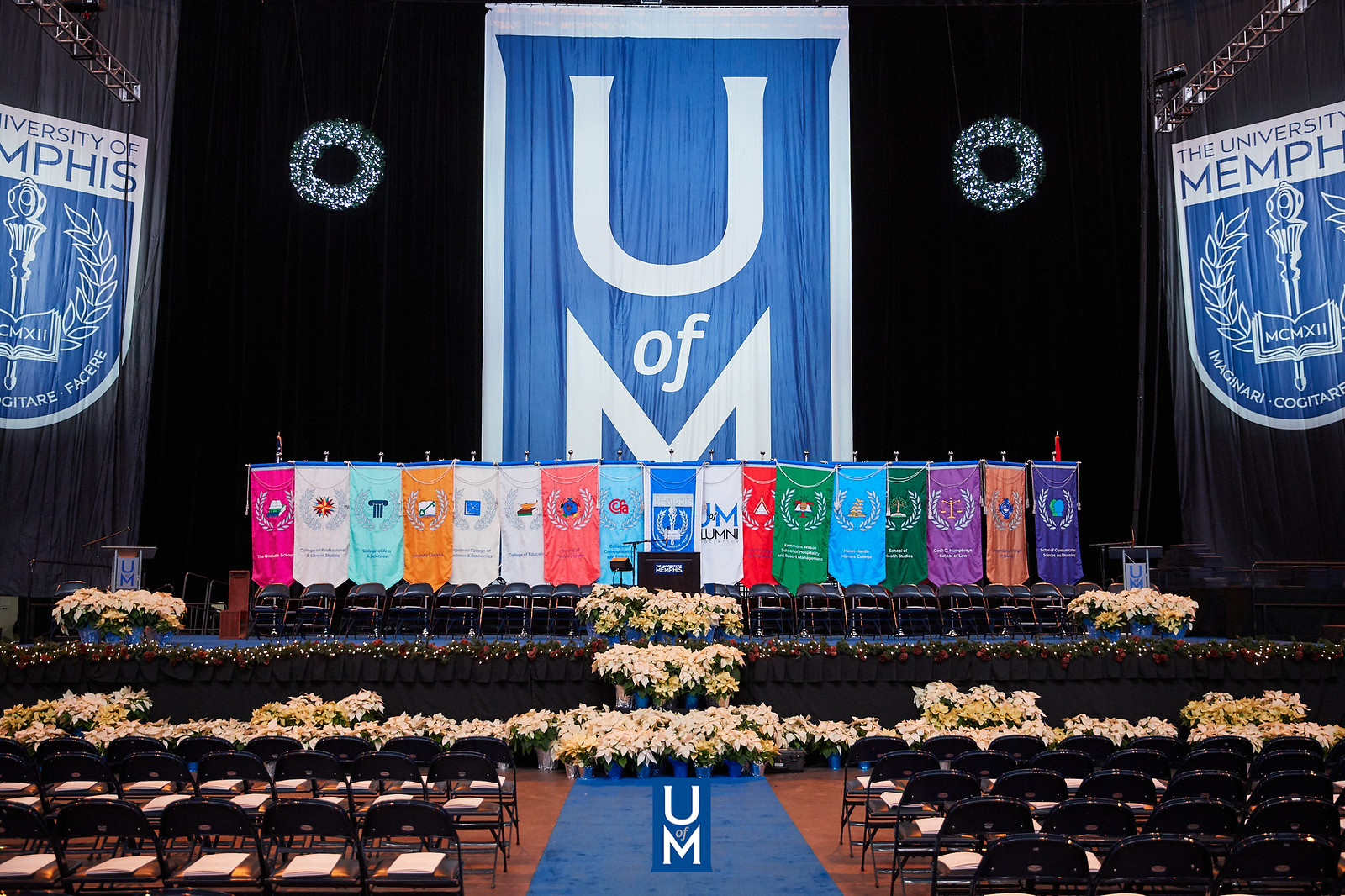
Electronic Theses and Dissertations
Identifier
1184
Date
2014
Document Type
Dissertation
Degree Name
Doctor of Philosophy
Major
Mathematical Sciences
Concentration
Applied Statistics
Committee Chair
E. Olusegun George
Committee Member
Dale Bowman Armstrong
Committee Member
Vinhthuy Phan
Committee Member
Stanley Pounds
Abstract
The last decade has ushered in an era of high dimensional, high volume data. In particular with the biotechnological revolution of the era, high-dimensional genomic studies of various designs have provided investigators with the tools to study thousands or even millions of genomic features simultaneously. These studies have shed new light on the underlying mechanisms of complex diseases. The accumulated knowledge of these complex relationships between genes has led scientists to formalize pathways and graphical networks that visually and succinctly give descriptions of the geometry of these relationships. With such knowledge, it has become possible to develop procedures for statistical inference, not just at the individual genes level, but at the more meaningful gene-set level. The focus of this thesis is the development of new statistical procedures for such gene-set analysis. After presenting an overview at the introduction, we give a comprehensive review of the literature relevant developments in the thesis in Chapter 2. In Chapter 3, we develop a Bayesian procedure that incorporates information contained in a gene graphical network, viewed as a directed graph, into the construction of prior distributions and we use the derived posterior distributions to construct statistical tests at the gene-set level. Our procedure extends the work of Pan (2006) and Wei and Pan (2008) which did not use the direction as information in the graphical network, but rather used undirected graphs and assumed a mixture model for the distribution to generate the posterior distribution of the mixing parameters via the use of a Markov random field. We demonstrate the gain in statistical power of our procedure over Pan and Wei's in an application to detect differentially expressed genes, and gene-sets by analyzing a data set that compares favorable risk and poor risk defined by cytogenetics in adults with acute myeloid leukemia (AML). To enhance comprehension of the vast and complex information in high-dimensional data from genomic studies, it is sometimes useful and desirable to have a procedure that relates such data to specific endpoints. In this regards, association tests are highly desirable. In Chapter 4, we propose a procedure which we label `Projection onto Orthogonal Space Testing (POST)' as a flexible method for testing association of gene sets and pathways with specific phenotypic endpoints while adjusting for other factors and variables as needed. In a simulation study, we demonstrate that POST has better operating characteristics than other methods recently developed to address the same objective. Thus we feel that POST does not only help to better understand treatment responses, but also prioritizes pathways for further study. We expect that POST will be especially valuable in clinical studies where cohorts with moderate to large sample sizes have rich high-dimensional data. Another new procedure for association testing which we label 'Locus Based Integrated Testing(LOCIT)' and an extension of the procedure -LOCITO- are introduced in Chapter 5. LOCIT is designed to test association of multiple forms of genomic data within a locus with an endpoint of interest in genomic studies. Given different forms of genomic data such as SNP genotypes, gene expression, and methylation levels, LOCIT performs one test per locus, taking several features at the locus into consideration. To illustrate the efficacy of LOCIT, we apply the procedure to a set consisting of SNP genotypes and gene profiling in an AML cohort to identify loci /genes that are associated with clinical outcomes. In chapter 6, we summarize our development of gene-set level association tests and outline future directions of our research in this area.
Library Comment
Dissertation or thesis originally submitted to the local University of Memphis Electronic Theses & dissertation (ETD) Repository.
Recommended Citation
Cao, Xueyuan, "Set Based Association Testing in High Dimensional Genomic Studies" (2014). Electronic Theses and Dissertations. 995.
https://digitalcommons.memphis.edu/etd/995
Comments
Data is provided by the student.