Taxonomy and Survey of Interpretable Machine Learning Method
Abstract
Since traditional machine learning (ML) techniques use black-box model, the internal operation of the classifier is unknown to human. Due to this black-box nature of the ML classifier, the trustworthiness of their predictions is sometimes questionable. Interpretable machine learning (IML) is a way of dissecting the ML classifiers to overcome this shortcoming and provide a more reasoned explanation of model predictions. In this paper, we explore several IML methods and their applications in various domains. Moreover, a detailed survey of IML methods along with identifying the essential building blocks of a black-box model is presented here. Herein, we have identified and described the requirements of IML methods and for completeness, a taxonomy of IML methods which classifies each into distinct groupings or sub-categories, is proposed. The goal, therefore, is to describe the state-of-the-art for IML methods and explain those in more concrete and understandable ways by providing better basis of knowledge for those building blocks and our associated requirements analysis.
Publication Title
2020 IEEE Symposium Series on Computational Intelligence, SSCI 2020
Recommended Citation
Das, S., Agarwal, N., Venugopal, D., Sheldon, F., & Shiva, S. (2020). Taxonomy and Survey of Interpretable Machine Learning Method. 2020 IEEE Symposium Series on Computational Intelligence, SSCI 2020, 670-677. https://doi.org/10.1109/SSCI47803.2020.9308404
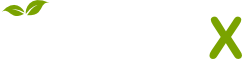
- Citations
- Citation Indexes: 14
- Policy Citations: 2
- Usage
- Abstract Views: 3
- Captures
- Readers: 40