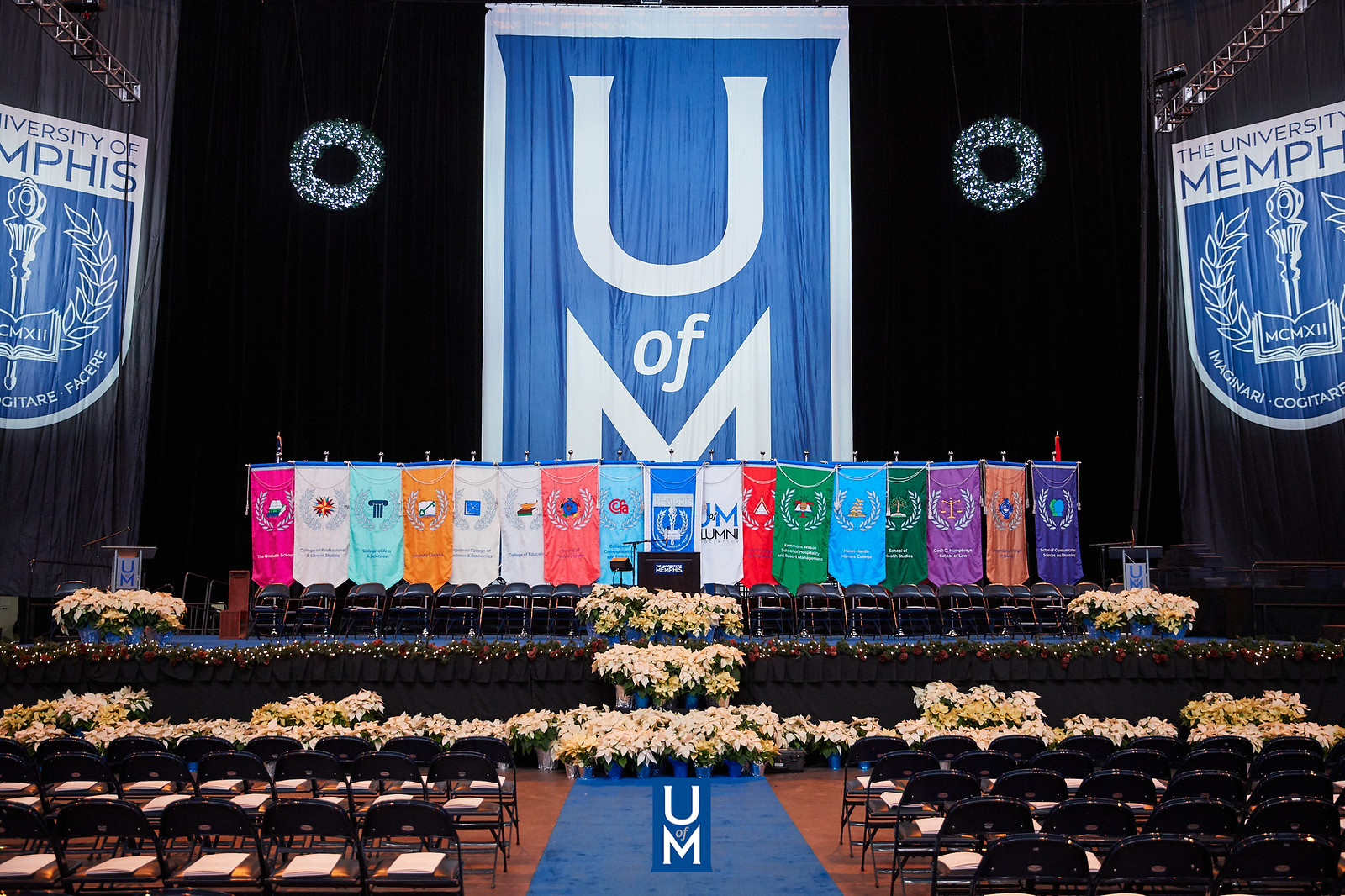
Electronic Theses and Dissertations
Identifier
23
Date
2010
Document Type
Dissertation
Degree Name
Doctor of Philosophy
Major
Computer Science
Committee Chair
Dipankar Dasgupta
Committee Member
King-Ip Lin
Committee Member
Vinhthuy Phan
Committee Member
Qishi Wu
Abstract
Many interesting immunological processes and mechanisms provide a potential source of inspiration for researchers to build Artificial Immune System (AIS), among which self versus nonself recognition is one of the distinctive mechanisms that are explored for AIS with minimum logical and technique overlap for any existing techniques. This dissertation explores a revised sense of "self" suggested in Pattern Recognition Receptors Model as a new immunity-based approach called Conserved Self Pattern Recognition Algorithm (CSPRA). The CSPRA alleviates the intrinsic limit of negative selection algorithms by combining the results of both self pattern recognition and negative selection for anomaly detection rather than simply assuming all foreign patterns are anomalies. To coordinate the detections from two signals in the CSPRA, both Classical CSPRA and Selective CSPRA are proposed. Classical CSPRA mirrors the immunological metaphor and does not trigger the costimulation of APC detector (signal 2) until the T cell detector suspects the encountered anomalous sample (signal 1), whereas Selective CSPRA exploits domain knowledge and randomized methods to resolve the conicts between two signals in the system. This dissertation demonstrates that the CSPRA has promising performance on breast cancer diagnosis and great potential in clinical diagnosis. Despite the articulation and clarification of the concept of conserved self pattern, a network-based Intrusion Detection (ID) model based on the CSPRA named CSPRA-ID, which is augmented with a novel schemeof near-deterministic detector generation, is presented. The CSPRA-ID worked very successfully on the DARPA network intrusion data. Multilevel Learning Algorithm (MILA) is the abstraction of the immunological events in humoral immunity with the features of multilevel recognition and online learning. The experiments have shown its success on anomaly detection. A MILA-based framework for automated and adaptive eye localization via face detection is proposed and implemented in this work. It applies a multilevel detection strategy resembling the top-down search to locate the eyes in face areas with the Viola-Jones object detection approach, prior to that the high-quality solutions are identified through the process of recognition and evolution. The experiments were performed with the PUT face database to demonstrate the strength and uniqueness of the proposed framework.
Library Comment
Dissertation or thesis originally submitted to the local University of Memphis Electronic Theses & dissertation (ETD) Repository.
Recommended Citation
Yu, Senhua, "Exploration of Sense of Self and Humoral Immunity for Artificial Immune Systems: Algorithms and Applications" (2010). Electronic Theses and Dissertations. 15.
https://digitalcommons.memphis.edu/etd/15
Comments
Data is provided by the student.