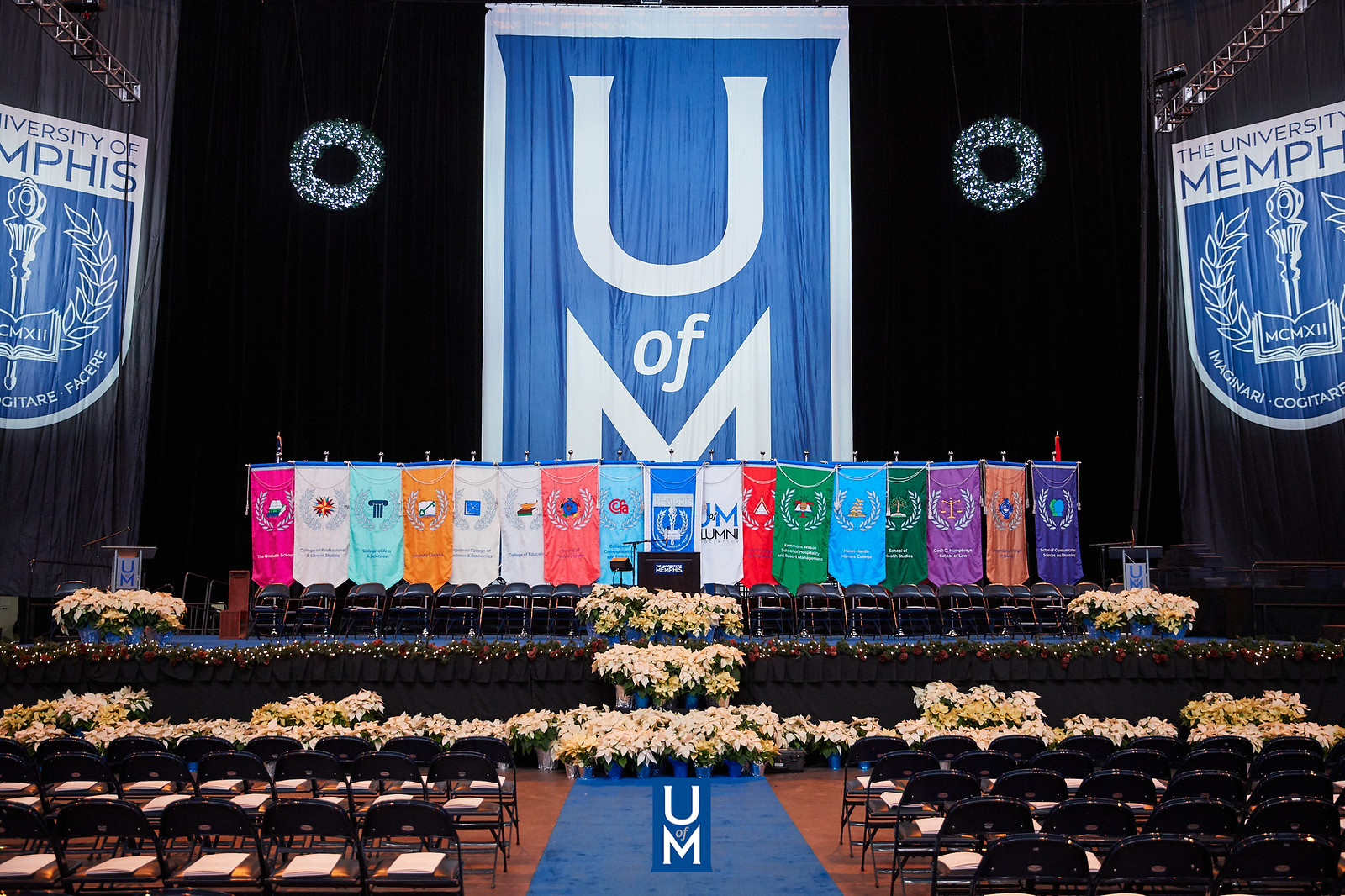
Electronic Theses and Dissertations
Identifier
6238
Date
2018
Document Type
Thesis
Degree Name
Master of Science
Major
Electrical and Computer Engr
Committee Member
Aaron Robinson
Committee Member
Eddie Jacobs
Committee Member
Madhusudhanan Balasubramanian
Abstract
Compressive Sensing (CS) has proven its ability to reduce the number of measurements required to reproduce images with similar quality to those reconstructed by observing the Shannon-Nyquest sampling criteria. By exploiting spatial redundancies, it was shown that CS can be used to denoise and enhance image quality. In this thesis we propose a method that incorporates an efficient use of CS to locate a specific object in zero-visibility environments. This method was developed after multiple implementations of dictionary learning, reconstruction, detection, and tracking algorithms in order to identify the shortcomings of existing techniques and enhance our results. We show that with the use of an over-complete dictionary of the target our technique can perceive the location of the target from hidden information in the scene. This thesis will summarize the previously implemented algorithms, detail the shortcomings evident in their outputs, explain our setups, and present quantified results to support its efficacy in the results section.
Library Comment
Dissertation or thesis originally submitted to the local University of Memphis Electronic Theses & dissertation (ETD) Repository.
Recommended Citation
Abu Hussein, Mohammed Eid, "Degraded Visual Environment Tracker" (2018). Electronic Theses and Dissertations. 1865.
https://digitalcommons.memphis.edu/etd/1865
Comments
Data is provided by the student.