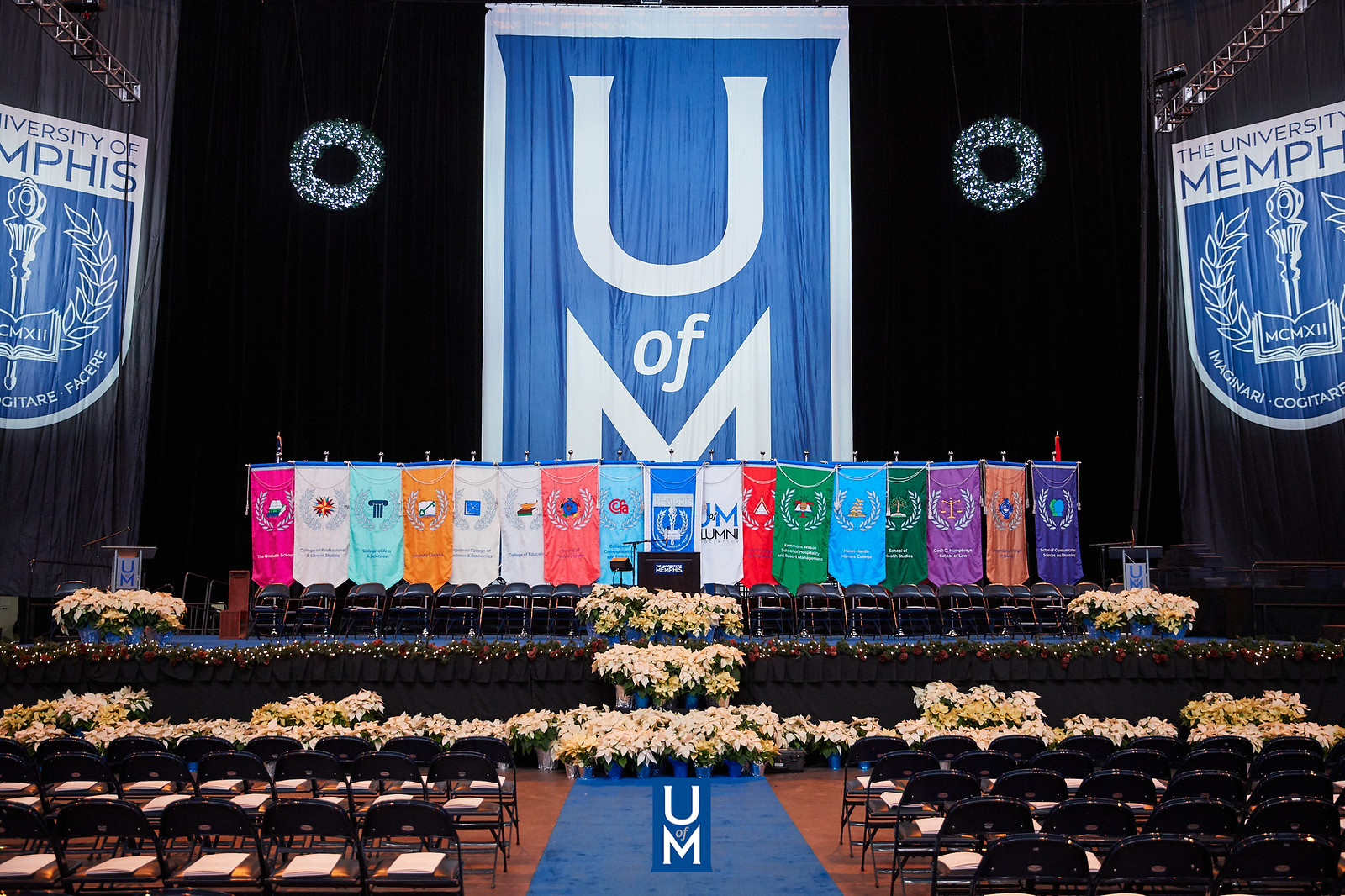
Electronic Theses and Dissertations
Date
2020
Document Type
Dissertation
Degree Name
Doctor of Philosophy
Department
Electrical & Computer Engineering
Committee Chair
Santosh Kumar
Committee Member
Eugene Buder
Committee Member
Madhusudhanan Balasubramanian
Committee Member
Pater Lau
Abstract
Stress is unavoidable in everyday life which can result in several health related short and long-term adverse consequences. Previous research found that most of the stress events occur due to interpersonal tension followed by work related stress. Enabling automated detection of stressful social interactions using wearable technology will help trigger just-in-time interventions which can help the user cope with the stressful situation. In this dissertation, we show the feasibility of differentiating stressful social interactions from other stressors i.e., work and commute.However, collecting reliable ground truth stressor data in the natural environment is challenging. This dissertation addresses this challenge by designing a Day Reconstruction Method (DRM) based contextual stress visualization that highlights the continuous stress inferences from a wearable sensor with surrounding activities such as conversation, physical activity, and location on a timeline diagram. This dissertation proposes a Conditional Random Field, Context-Free Grammar (CRF-CFG) model to detect conversation from breathing patterns to support the visualization. The advantage of breathing signal is that it does not capture the content of the conversation and hence, is more privacy preserving compared to audio. It proposes a framework to systematically analyze the breathing data collected in the natural environment. However, it requires wearing of chest worn sensor. This dissertation aims to determine stressful social interaction without wearing chest worn sensor or without requiring any conversation model which is privacy sensitive. Therefore, it focuses on detecting stressful social interactions directly from stress time-series only which can be captured using increasingly available wrist worn sensor.This dissertation proposes a framework to systematically analyze the respiration data collected in the natural environment. The analysis includes screening the low-quality data, segmenting the respiration time-series by cycles, and develop time-domain features. It proposes a Conditional Random Field, Context-Free Grammar (CRF-CFG) model to detect conversation episodes from breathing patterns. This system is validated against audio ground-truth in the field with an accuracy of 71.7\%.This dissertation introduces the stress cycle concept to capture the cyclical patterns and identifies novel features from stress time-series data. Furthermore, wrist-worn accelerometry data shows that hand gestures have a distinct pattern during stressful social interactions. The model presented in this dissertation augments accelerometry patterns with the stress cycle patterns for more accurate detection. Finally, the model is trained and validated using data collected from 38 participants in free-living conditions. The model can detect the stressful interactions with an F1-score of 0.83 using stress cycle features and enable the delivery of stress intervention within 3.9 minutes since the onset of a stressful social interaction.
Library Comment
Dissertation or thesis originally submitted to ProQuest
Recommended Citation
Bari, Rummana, "Detecting Stressful Social Interactions Using Wearable Physiological and Inertial Sensors" (2020). Electronic Theses and Dissertations. 2442.
https://digitalcommons.memphis.edu/etd/2442
Comments
Data is provided by the student.