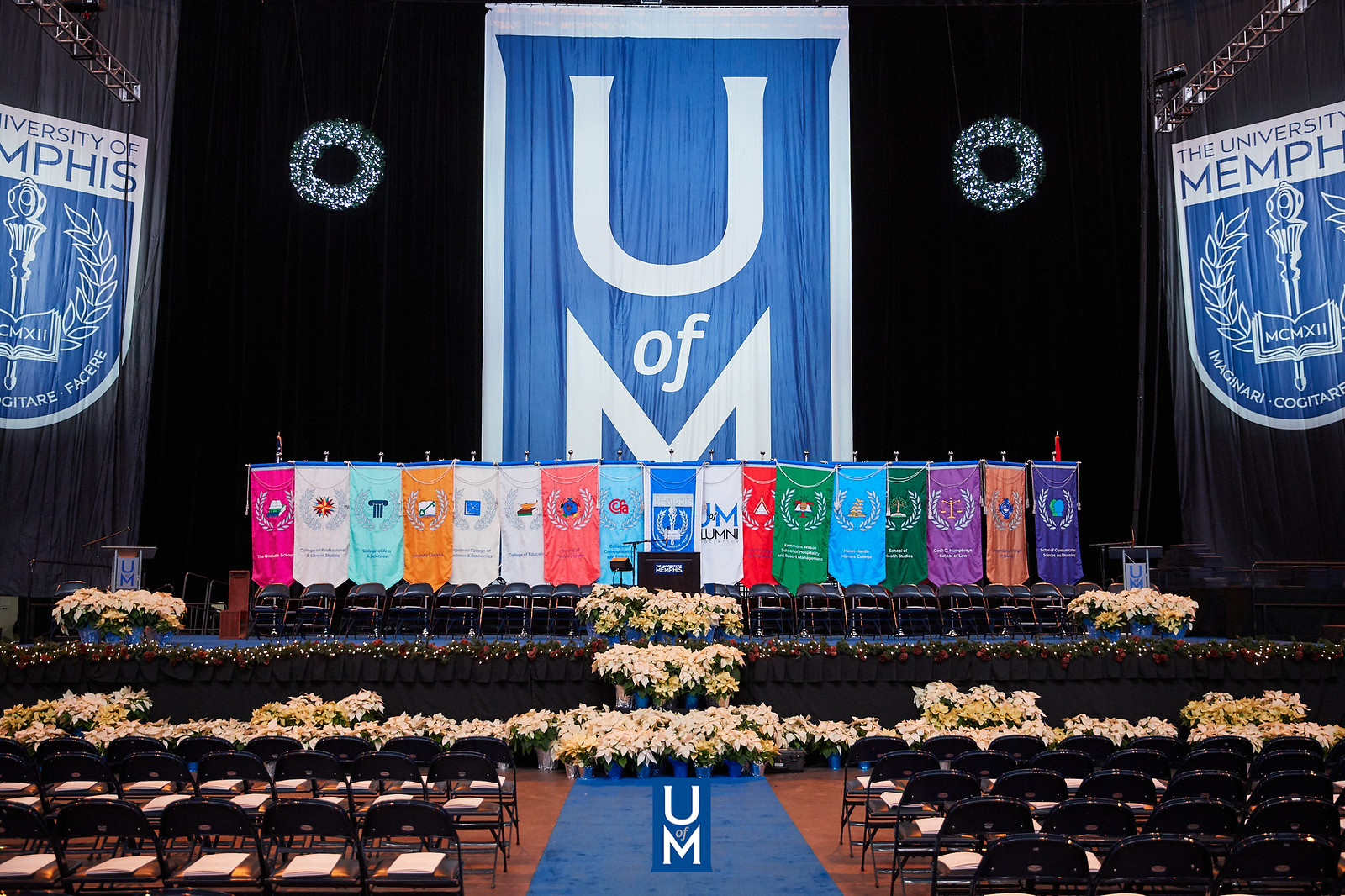
Electronic Theses and Dissertations
Date
2020
Document Type
Dissertation
Degree Name
Doctor of Philosophy
Department
Electrical & Computer Engineering
Committee Chair
Mohammed Yeasin
Committee Member
Eugene Eckstein
Committee Member
Madhusudhanan Balasubramanian
Committee Member
Faruk Ahmed
Abstract
Osteoarthritis (OA) is the foremost chronic and degenerative joint disorder. Early detection and risk assessment of OA is critical to prioritize proper patient care due to limited treatment options. Our main goal is to develop techniques to characterize and assess OA severity for any joints (i.e., Knee, Hip, lumbar spine, hands, and cervical spine) and building a decision support system (DSS) to assist clinical practitioners. This dissertation focuses only on the Knee Osteoarthritis (KOA) as it is the most prevalent OA type.Generally, the assessment of KOA is performed using radiographs and patient clinical records. Expert assessments are based upon inadequate quality radiographs and medical records containing a broad range of highly non-correlated variables with a high percentage of missing values. To address a multitude of issues involving KOA assessment, we present several data-driven, parameter optimized, and interpretable models using the entire cohort of osteoarthritis initiative (OAI) dataset.First, we focus on detecting and localizing the joint under consideration for OA severity assessment from radiographs. We modify established localization deep learning-based models to account for variances resulting from different imaging devices and imaging poses such as scale and aspect ratio. Second, we develop parameter optimized and explainable deep learning-based models for classifying the OA severity using the KellgrenLawrence (KL) scale of KOA from radiographs. We develop a sequential computational pipeline for training and testing using Bayesian hypermeter optimized architecture to ensure maximum performance with better generalization.Third, to demonstrate the explainability, verify learning, and decode assessment failure, we develop novel and class-independent Eigen-CAM to visualize learned patterns of developed models for OA severity assessment based on radiographs. Fourth, to find alternative sources of data complementary to radiographs in building models, we explore the patient clinical records that have received insignificant attention from practitioners as well as the modelers. We perform data imputation using MissForest; address class imbalance by under-sampling the majority class; select most relevant features consistent over a range of model parameters using Stability Selection, and implement a number of methods such as Random Forests (RF), Epsilon-support vector regressor (Epsilon-SVR) and Elastic Net (EN) to predict KOA severity.
Library Comment
Dissertation or thesis originally submitted to ProQuest
Notes
embargoed
Recommended Citation
Bany Muhammad, Mohammed Adel, "Interpretable and Optimized Models for Osteoarthritis Assessment" (2020). Electronic Theses and Dissertations. 2883.
https://digitalcommons.memphis.edu/etd/2883
Comments
Data is provided by the student.