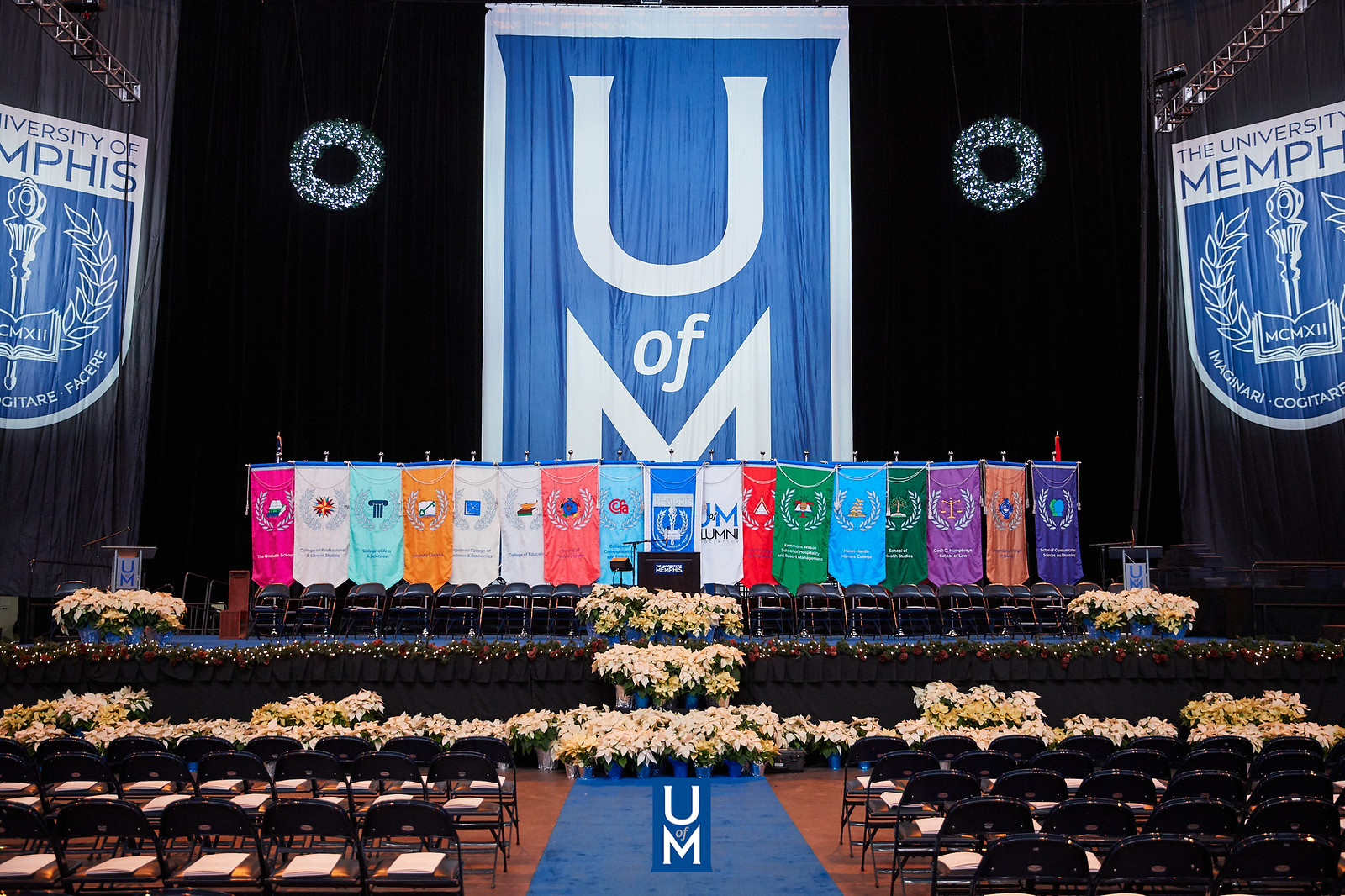
Electronic Theses and Dissertations
Date
2023
Document Type
Thesis
Degree Name
Master of Science
Department
Electrical & Computer Engineering
Committee Chair
Chrysanthe Preza
Committee Member
Chrysanthe Preza
Committee Member
Aaron Robinson
Committee Member
Eddie Jacobs
Abstract
Hyperspectral unmixing is the process of decomposing mixed pixels into distinct spectra or endmembers. In the hyperspectral unmixing process, a linear mixture model and the Fully Constrained Least Square approach are used to estimate an abundance map, which highlights the amount of each endmember that is present in the scene. This unmixing process is essential to tasks, such as target detection, where a reference endmember is provided, and the goal is to highlight areas in the scene that correspond to that reference endmember. As the number of endmembers is important to the unmixing process, we conducted an investigation in which we found that denoising a hyperspectral image, can cause the estimation of the number of endmembers to be inflated by 5-6 times, indicating the importance of estimating the number of endmembers before applying image denoising. In addition, hyperspectral unmixing could be used to identify materials in the scene, and as part of this thesis we conducted a study using the Samson dataset, in which the extracted endmembers were compared to their ground truths counterparts, both visually and using spectral metric called SID. The visual similarity between extracted endmembers and the ground truth, and the minimal spectral deviations between them are indicators of a successful unmixing. Hyperspectral unmixing can also aid in improving the task of anomaly detection, and this thesis presents an ensemble anomaly detector that integrates abundance maps estimated through hyperspectral unmixing, to outperform state of the art anomaly detectors, and offer the ability to generalize on different images. Furthermore, since the ensemble method consists of multiple sub-methods, then any improvements in those sub-methods will contribute to improving the overall performance of the ensemble method. This thesis has made efforts to enhance the target abundances in the experimental “Arizona dataset” using image processing techniques, such as Gaussian Blur and Sobel Edge enhancement. We show that these methods improved anomaly abundances, as evidenced by enhanced F1 scores.
Library Comment
Dissertation or thesis originally submitted to ProQuest.
Notes
Open Access
Recommended Citation
Younis, Mohammed, "INVESTIGATION OF HYPERSPECTRAL DATA UNMIXING AND ITS IMPACT ON THE TASK OF ANOMALY DETECTION" (2023). Electronic Theses and Dissertations. 3343.
https://digitalcommons.memphis.edu/etd/3343
Comments
Data is provided by the student