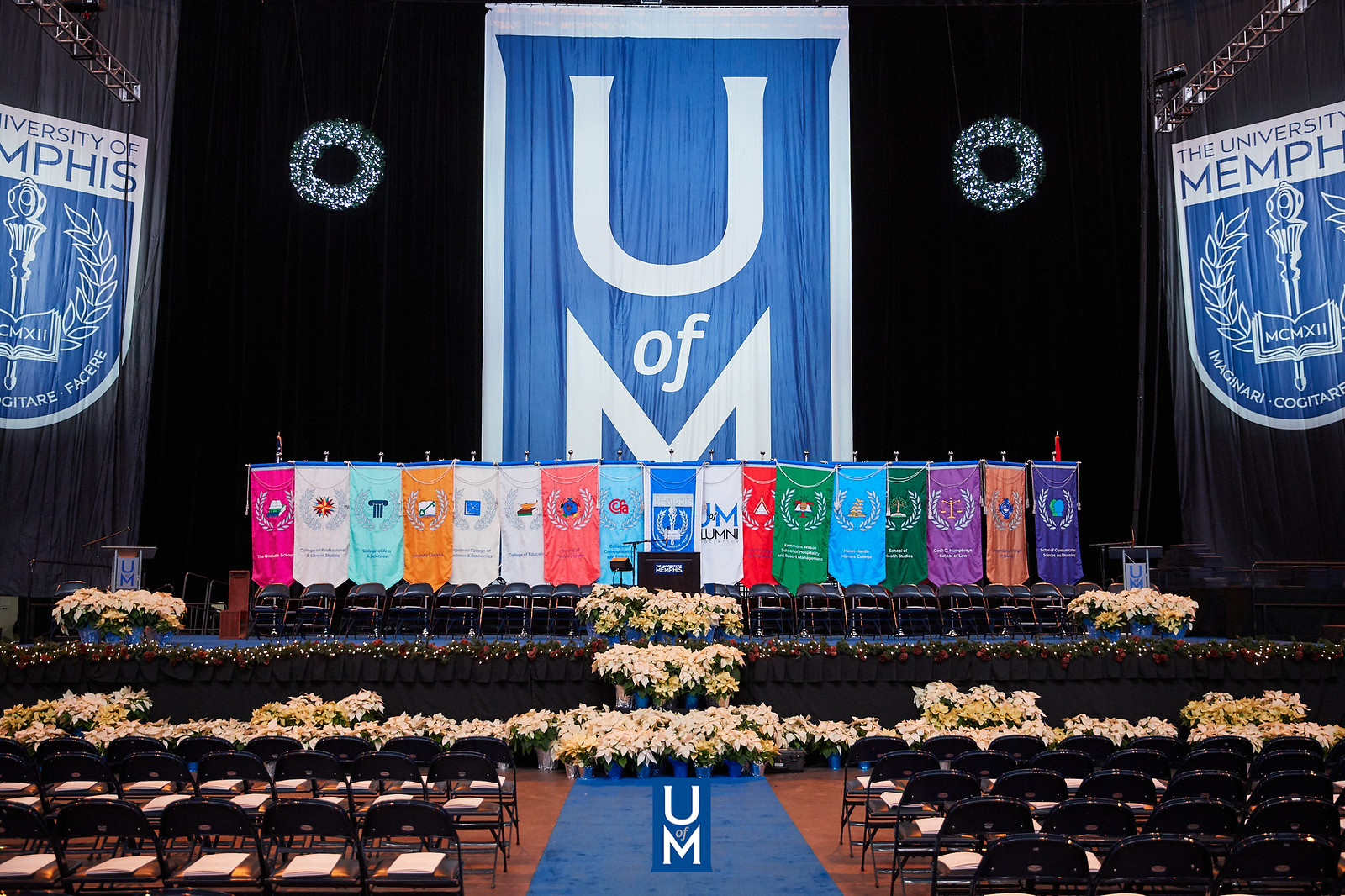
Electronic Theses and Dissertations
Date
2023
Document Type
Thesis
Degree Name
Master of Science
Department
Physics
Committee Chair
Xiao Shen
Committee Member
Thang B Hoang
Committee Member
Francisco M Sanchez
Abstract
Neural activity correlates with a wide variety of phenomena relating to the animal originating the activity. It is possible to exploit those correlations by measuring the activity and mathematically transforming it via a so-called decoding process to determine what, physically, the activity represented. The signals measured are typically nonlinear, and though classical statistical techniques exist for handling these signals, increasingly influential methods including neural networks and the Koopman operator formalism provide new avenues to explore for handling such nonlinear problems. We propose a method of decoding utilizing a neural network representation of the Koopman operator and test it on the task of decoding a rat’s physical position from the activity of place cells in its hippocampus. We find that although it is not yet competitive with existing methods, the technique has merit and can be improved.
Library Comment
Dissertation or thesis originally submitted to ProQuest.
Notes
Open Access
Recommended Citation
Edwards, Kyle, "Evaluation of Koopman Operator Neural Decoding Scheme" (2023). Electronic Theses and Dissertations. 3347.
https://digitalcommons.memphis.edu/etd/3347
Comments
Data is provided by the student